AI & the Future of Assessment in Higher Education
Recently I published this video to my youtube channel, summarising some of the issues that ChatGPT introduces to assessment in higher education. Since then, in engaging with colleagues in a wider discussion around generative AI in DCAD and beyond, some of my (our) thinking has developed. I want to use this blogpost to give a broad summary of these ideas, linking to some further resources.
AI is Here.
Like it or not, Artificial Intelligence (AI) is playing an increasing role in our everyday lives. Machines can be trained to perform a wide variety of tasks, such as recognising speech, natural language processing, object detection, image classification and sentiment analysis. We interact with these systems on a daily basis, from the recommendation systems in YouTube, Netflix, Amazon and Spotify, to virtual assistants such as Alexa, Google Assistant or Siri, to the Chatbots in customer services, to the personalisation of content and targeting of advertisements. Many industries are already harnessing the power of AI, such as: fraud detection in finance; predictive modelling in healthcare, manufacturing and transportation; and the optimisation of supply chains and logistics. In this way, we might consider AI to be ‘ubiquitous’, embedded in our interactions with the world in a way that has already become ‘invisible’.
To this extent, AI has also quietly been taking an increasing role in HE. Many students are users of services such as Grammarly, an AI typing assistant that can not only review spelling, punctuation, and grammar, but also provide suggestions around clarity, engagement, tone and style. Likewise, the internet searches we rely on to source academic content use complex AI models to rank the most relevant content in relation to search queries. To these ends, the incorporation of AI in HE is not some distant future, it is already here (note the tweet from Mike Sharples below is from May 2022!). There are many AI applications HE is beginning to explore, such as adaptive learning systems, analysis of student scores and assessment to identify patterns and trends, or chatbots which could improve the student experience by providing quick, accurate information and support.
It is in this context we should view the ‘hype’ around the recently released ChatGPT. This is a form of generative1 (the ‘G’ in ‘GPT’) AI, which generates coherent and fluent human-like responses in response to a function call (or prompt). This is often seen as ‘convincing’ sounding text can be difficult to distinguish from those written by a human, particularly on first glance. The model is pre-trained (the ‘P’ in ‘GPT’) on a vast dataset of text from the internet (reported to be 300 billion words or 570GB of data). It uses a type of neural network called a Transformer model (the ‘T’ in ‘GPT’) to understand context, generate new text, and answer questions in a human-like manner. It can be understood as a Large Language Model. It has been developed by OpenAI and is built on GPT-3 (Generative Pre-trained Transformer)2.
Concerns & Opportunities
The primary concern that has arisen around generative AI3 in HE is around student assessment, specifically that a student would be able to type the essay question as a prompt, and the model would be able to produce something ‘passable’ with minimal effort or understanding of the topic. There are also further limitations of AI, such as AI bias. An AI system will inherit the biases present in the data it is trained on, as well as from the humans who have provided any supervised training4, for example facial recognition systems have been widely shown to have a gender and racial bias. Some have reported ChatGPT to be ‘rife with bias’, and can replicate similar gender and racial biases despite OpenAI putting certain controls on the prompts that can be passed. While the full dataset that ChatGPT has been trained on has not been made public, it is fair to assume that it will replicate the broad biases across the web which is predominantly written by white men in western countries. The widespread adoption of tools such as generative AI, if not used critically, means that these biases could be perpetuated.
Can we detect AI writing?
There are currently certain tasks that generative AI is less good at. Many have noted factual inaccuracies in the responses generated by ChatGPT, which are particularly noticeable if you are a domain expert in the topic. There are concerns about misinformation if it is considered a point of ‘truth’. Likewise, ChatGPT also often invents convincing sounding references to back up its argument, particularly journal articles (although it has also been seen to identify key literature well). With minimal prompts, the output can be generic and descriptive, with no local knowledge of the course or data associated with it (unless this is inputted to the model as a prompt by the user). It is also less convincing at generating new or novel arguments, something that might be considered ‘truly’ original or creative. The nature of generative AI means it is always calculating the probability of the next word generated, therefore longer pieces of writing are less likely to have a sense of throughline or argument. Longer pieces of writing (1000 words+ or so) also appear more challenging to generate in ‘one go’.
While these features might be considered ‘smoking guns’ in identifying a response generated by ChatGPT specifically, it is paramount to mention that these limitations exist at the time of writing and it is inevitable that these tools will only improve over time. Any shortcomings can be easily circumnavigated, for example, by checking and correcting references, feeding in additional information (e.g. details of a placement), or generating an essay a paragraph at a time.
A certain excitement has arisen in some areas of academia around apps that ‘detect’ ChatGPT writing. This, however, comes with significant problems. Phillip Dawson raises several legal and ethical challenges around such Apps, and concern around reports of false positives, privacy, accuracy and robustness. Such ‘detectors’ can also be easily circumnavigated through paraphrasing tools available to students such as Quillbot, Grammarly or Paraphraser.io, so they are undetectable. Arbitrary percentage markers for (any) originality reports are likely to be ill-judged, and perpetuate inequality, favouring students who are ‘in the know’ about how to circumnavigate them.
Can we harness the power of AI in teaching and learning in HE?
It is essential to recognise the impressive abilities of these generative AI models. Users have found ChatGPT excels particularly at report writing, structuring content in a logical and coherent fashion. It is also particularly good at summarising content concisely, excelling particularly at short answer questions or generating recommendations. It can often define and summarise ideas well, particularly in writing that is more procedural, so can be utilised effectively to identify technical language or generate effective turns of phrase. It can also produce more sophisticated pieces of writing through iterations. For example, a skilled and knowledgeable user could repeatedly iterate on a paragraph to improve the clarity and flow, or even incorporate certain authors into an argument or reflective piece.
If used well, generative AI could be a powerful tool for students to improve their academic work. These models could be a useful tool for educators, in terms of structuring teaching or learning design, incorporating it into more interactive and engaging learning materials, providing more personalised instruction and immediate feedback. These tools could even be used to help mark assignments and exams, providing more ‘accurate’ and consistent evaluations, reducing subjectivity across markers (and hence have the potential to reduce complaints about ‘generous’ or ‘harsh’ markers).
But what about academic integrity?
While it would be clearly problematic for a student (or staff) to ‘copy-paste’ a response from a generative AI model with no understanding of the content, Foteini Spingou notes that with appropriate (and ‘up to date’) instructor awareness these students are likely to ‘get caught’ or a ‘bad’ mark. Principles of academic integrity still apply – copying and pasting, or poorly paraphrasing large swathes of text from any external source, particularly without attribution, is not and has never been considered sound academic practice.
Educators can also use generative AI output in more creative ways (see Ryan Watkins’ advice on updating your course syllabus for ChatGPT), such as writing a critical reflection around the model’s output. Moreover, the use of AI tools like ChatGPT is becoming increasingly prevalent in various industries, and as such, it is important for students to learn how to use them so as to be competitive in the job market. Having knowledge and skills in AI technologies can open up a wide range of job opportunities in fields such as natural language processing, computer vision, and machine learning. Being familiar with AI tools can also give students an advantage in other (emerging) industries that are beginning to integrate AI into their operations. By incorporating AI tools like ChatGPT into their teaching and learning practice, educators can modernise their practice, making their teaching and learning more relevant to the modern world, helping their students to prepare effectively for the job market.
How should we respond?
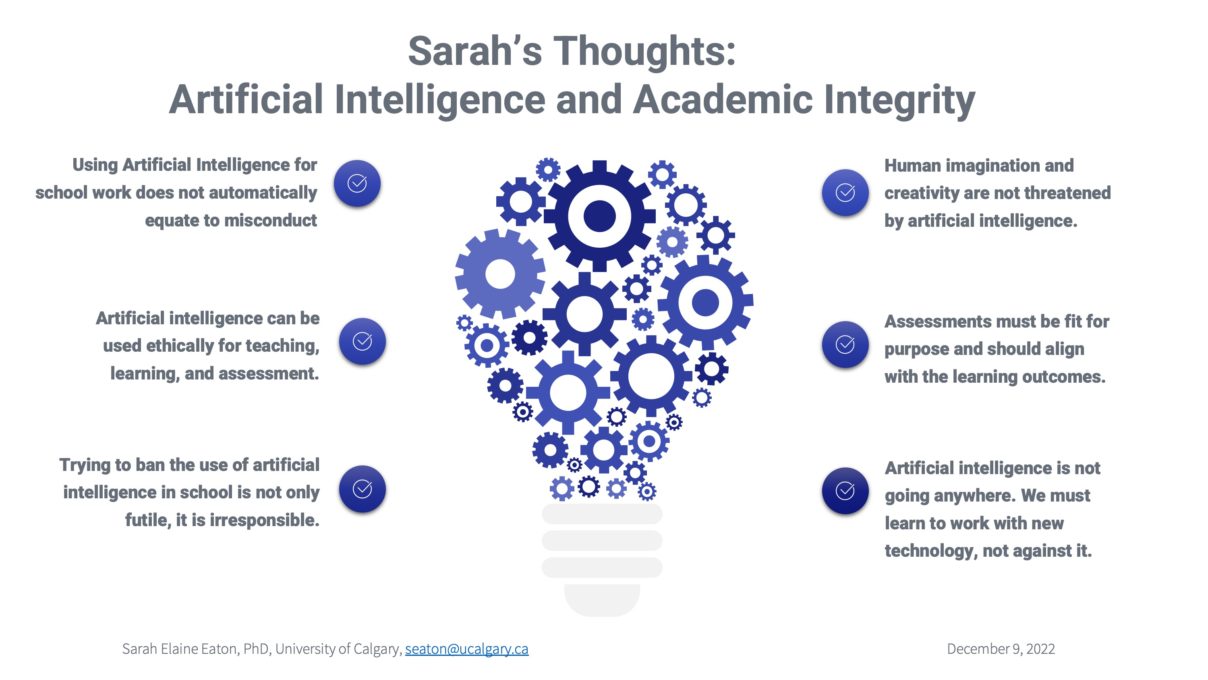
Embrace
There is no option but for HE to embrace this technological advancement, as we have done in the past with technologies such as the pocket calculator, word processing, and search engines. Used effectively, AI can enhance student and staff practice, making a more engaging and personalised experience for students based on their individual learning needs and progress. Generative AI can optimise both student and staff activities. If used effectively, generative AI can help improve upon academic work, helping reach learning objectives more effectively and efficiently, allowing students and staff to concentrate on the most meaningful learning activities. The tools that are available to students (and have been for some time) mean we are already moving to a position of AI-enhanced education, and it would be futile to interrupt this course of action.
Educate
While it is undoubtedly important that educators are aware of what generative AI is capable of, Andrew Piper notes that ChatGPT is a novel technology for students too. While some may be able to use it well, many will not know much about it, how to use it or when to trust it. To these ends we must engage in an open and honest discussion with our students about ChatGPT. We certainly cannot hide it from them, nor ban its use. We should use these tools to engage in wider discussions about academic integrity in our own disciplines, as implications are likely also to be disciplinarily specific.
Enhance
The prevalence of these tools should be a catalyst for diversifying assessment, as well as teaching and learning. If educators feel an assessment could be answered just as well (or better) by generative AI, it is perhaps a prudent time to evaluate their assessment and/or learning objectives – ChatGPT may force us to make changes to assessment we should have been making anyway. While there may be limited instances where in-person exams are appropriate to ensure understanding of a topic, our focus should be on authentic assessment (see Sally Brown and Kay Sambell’s excellent work around this, for example), addressing skills that are relevant to the modern world. AI provides us with enticing opportunities to enhance creativity and criticality, be that the redesign of learning materials or a consideration for the role of AI in society at large. It is imperative for HE to engage with this if it is to remain relevant, and essential in preparing the workforce of the future.
Conclusions
AI is already integral to modern society, and generative AI tools such as ChatGPT are here to stay and will only improve. AI tools are not new in HE, and to these ends our focus should be on how we move forward towards the emerging path of AI enhanced education for learning, teaching and assessment. While detection solutions may have some value in identifying common patterns of AI generated text, they are also highly problematic ethically and practically.
By showing students how to appropriately utilise generative AI, we can help build their digital literacy and engagement, making their learning more relevant to the modern world. AI provides us with enticing opportunities to enhance creativity and criticality, and it is imperative for HE to engage to remain relevant, and essential in preparing the workforce of the future.
Footnotes
- Generative AI models are trained on a large dataset of existing data and can generate new, previously unseen data that is similar to the training data.
- Midjourney and DALL-E 2 are also forms of generative AI, which use image rather than text-based processing. Image based generative AI may have separate / related implications, but for the purposes of this blogpost ‘generative AI’ refers predominantly to text-based models.
- ChatGPT is currently being used synonymously with generative AI, but we should consider this in relation to the many other tools which utilise the same or similar technology, including but not limited to: Jasper Chat; Quattr.com; ChatSonic and Perplexity.ai. Various other ‘tech giants’ are also working on GPT alternatives, including: LaMDA, BERT, Transformer-XL, XLNet, ALBERT, T5 and ELECTRA by Google; RoBERTa by Facebook; CTRL by Salesforce; Megatron by NVIDIA. The successor to GPT-3, GPT-4, is also widely rumoured to be released soon. We must therefore consider ChatGPT in the context of other tools we can broadly call ‘Generative AI’, both in what is available now and is likely to be available in the short-, medium- and long-term future.
- Transformer models are trained predominantely using a form of unsupervised learning, in that the model learns the underlying structure of the data, such as patterns, clusters, or probability distributions with more minimal human input (other than selecting/cleaning up the data it is trained on). In contrast, supervised learning uses labelled data, where ‘human bias’ may be more of a factor to contend with. While it is not known exactly how ChatGPT is trained, current thinking suggests that GPT-3 was ‘fine tuned’ with human trainers which fed back dynamically in a series of machine learning models (a form of Reinforcement Learning through Human Feedback or RLHF). See this video from Computerphile for more detail: ChatGPT with Rob Miles.
Further Resources
Curated Resources for ChatGPT
Learning that Matters | Cynthia Alby | Breaking News: ChatGPT breaks higher ed
Bryan Alexander | Resources for exploring ChatGPT and higher education
Anna Mills | AI Text Generators Sources to Stimulate Discussion among Teachers
ChatGPT – game over (education) player one? | Malcolm Murray (available only internally to Durham University)
Want to know more about AI?
The MIT Deep Learning and Artificial Intelligence Lectures are an excellent introduction for a range of topics around AI. I found the video on Deep Learning Basics particularly helpful in getting the ‘broad strokes’ around AI.
The 3Blue1Brown series on Neural Networks are an excellent introduction to the mathematics involved in basic neural networks – this is the bedrock for more complex neural networks (such as Transformer models like ChatGPT).
The YouTube channel computerphile also has some excellent explainer videos around AI, such as this series on neural networks & videos on How AI Image Generators Work, AI Language Models & Transformers & GPT-3.
About the Author(s)
Matt Wood is a Digital Learning Developer at DCAD, Durham University. This blogpost was written in conversation with many colleagues at DCAD, in particular Nic Whitton in preparing papers for the University, Sam Nolan and Malcolm Murray.
Last updated 2 Feb 2023 – amendment around the nature of supervised/unsupervised learning